Forecasting in the Age of Chaos: Why Every Model Needs an Escape Plan
- Alex Pickworth
- Feb 23
- 3 min read
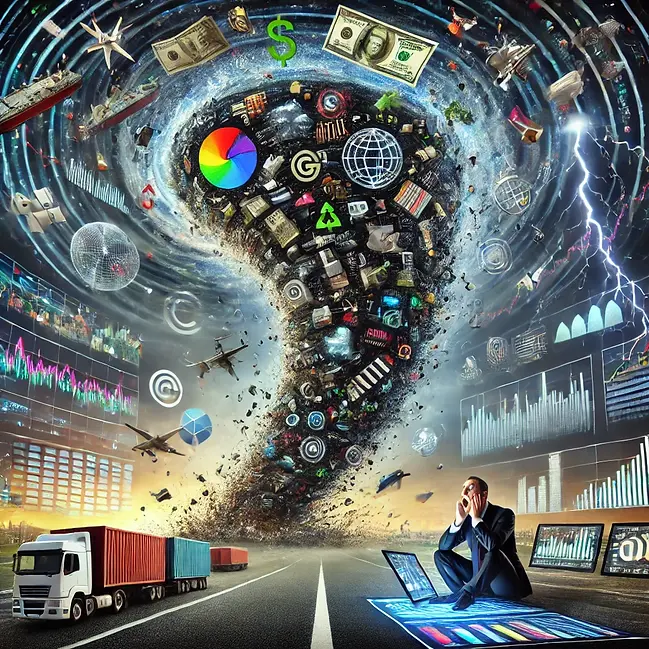
During a media mix modeling (MMM) development session, a C-Suite client once asked me, "When will we be done?" Without hesitation, I replied, "NEVER." While my response was cheeky, it underscored a critical truth: in today's volatile environment, modeling is an ongoing process. Competitors continually enhance their models, and to remain competitive, we must do the same. Evolution isn't optional; it's a business imperative. Ceasing to evolve equates to obsolescence.
The New Normal: Unprecedented Uncertainty
In the past nine weeks alone, we've witnessed a series of events that have upended traditional market assumptions:
Market Volatility: The Dow Jones Industrial Average plummeted 700 points in a single day, marking the worst downturn of 2025, fueled by fears over economic growth.
Automotive Industry Disruptions: Tesla experienced a staggering 63% drop in sales in France, the EU's second-largest electric vehicle market, highlighting the fragility of even leading innovators.
Consumer Behavior Shifts: A significant number of Canadians are now opting to replace American products, especially groceries, reflecting changing consumer sentiments and potential trade tensions.
Trade Policy Turbulence: President Trump's recent focus on value-added tax (VAT) policies has intensified trade stand-offs, introducing new layers of complexity to international commerce.
These events exemplify the rapid and unpredictable shifts in the global economy, rendering static models obsolete.
Key Arguments
The Perils of Overreliance on Historical Data
Traditional models often operate under the assumption that historical data is a reliable predictor of future outcomes. However, recent events have demonstrated that past performance is not indicative of future results. For instance, Tesla's unexpected sales decline in France challenges the notion that market leaders are immune to sudden downturns.
Technical Insight: Models must incorporate real-time data feeds and adaptive algorithms that can recalibrate in response to emerging trends, rather than relying solely on historical datasets.
Incorporating Contingency Protocols
Every major shock is often dismissed as an outlier, but in today's world, anomalies have become commonplace. If your forecasting model lacks built-in contingencies to override outdated assumptions and switch to survival mode, it's not a model—it's a delayed reaction.
Technical Insight: Implementing Bayesian time-varying coefficient models allows for parameters that adjust dynamically in response to new data, providing a framework for models that can "learn" and adapt to unforeseen events.
Prioritizing Flexibility Over Illusory Accuracy
In a world where a single policy change can erase billions in market value overnight, what's more valuable: a model that was 95% accurate last year, or one that's 80% accurate but adapts in real-time? The future belongs to models that don't just predict change—they expect it.
Technical Insight: Employing ensemble methods, such as gradient boosting machines, can enhance model robustness by combining multiple algorithms to offset individual weaknesses, leading to more resilient predictions in volatile environments.
The Role of Advanced Modeling Techniques
To navigate this era of uncertainty, consider integrating the following approaches:
Neural Networks and Deep Learning: These models excel at identifying complex, non-linear patterns in large datasets, making them invaluable for capturing subtle shifts in consumer behavior or market dynamics that traditional models might overlook.
Rolling Window Models: By continuously updating the dataset to include only the most recent observations, these models ensure that forecasts reflect the current state of the world, enhancing relevance and responsiveness.
Regime-Switching Models: These allow for different sets of parameters depending on the prevailing market conditions, enabling the model to switch between "regimes" in response to structural changes, such as sudden economic shocks or policy shifts.
Markov Chain Monte Carlo (MCMC) and Variational Inference: These statistical methods provide robust frameworks for estimating the uncertainty and variability in model parameters, facilitating more informed decision-making under uncertainty.
Conclusion
In an era where unpredictability is the only constant, clinging to static models is a recipe for obsolescence. By embracing adaptive modeling techniques and acknowledging the limitations of traditional approaches, businesses can not only navigate the chaos but also find opportunities within it. Remember, the objective isn't to predict the future with unerring accuracy—it's to build systems resilient enough to withstand the unforeseeable and flexible enough to adapt swiftly. Evolution in modeling isn't just a strategy; it's a survival imperative.
留言